First the data, then AI: How Trensition Came To Life
Artificial intelligence has become a dominant force in society in the last decade thanks to significant technological breakthroughs. This has resulted in an explosion of companies offering new AI-based applications.
AI is coming of age
In the last decade or so, AI evolved from being just one of the many promising technologies to a dominating world force that is rapidly transforming every imaginable aspect of our society. Its success is due to the convergence of some relatively recent major scientific and technological breakthroughs that made applying AI in the real world possible.
This resulted in a true explosion of new companies creating and offering AI-based applications to support or automate human tasks.
Trensition has been one of these companies since 2019. At our company, we built an AI-driven strategic intelligence platform to support managerial decision-making in organizations.
AI & Data Management
Building commercially viable AI solutions requires expertise in various domains, ranging from fundamental research to marketing and everything in between. But there is, in my opinion, only one aspect that stands out in the entire building process: data management.
It doesn't matter how advanced, efficient, or fast your algorithms are; if data management is not part of your company's core processes, there is a chance you will be competed out of the market by someone with better data (quality) and less fancy algorithms.
So, what does it mean to manage data as an AI company? Drawing from our experiences, decisions, failures, and successes since our establishment in 2019 and the research period from 2017 and 2019, I will delve into this topic.
First, I will discuss how and why you should apply data management practices in the very early stages of your company. Then, I will focus on our experiences at Trensition regarding this matter.
Read: Trensition's Moonshot Thinking: Making the Future Less Uncertain
Early-stage Data Management
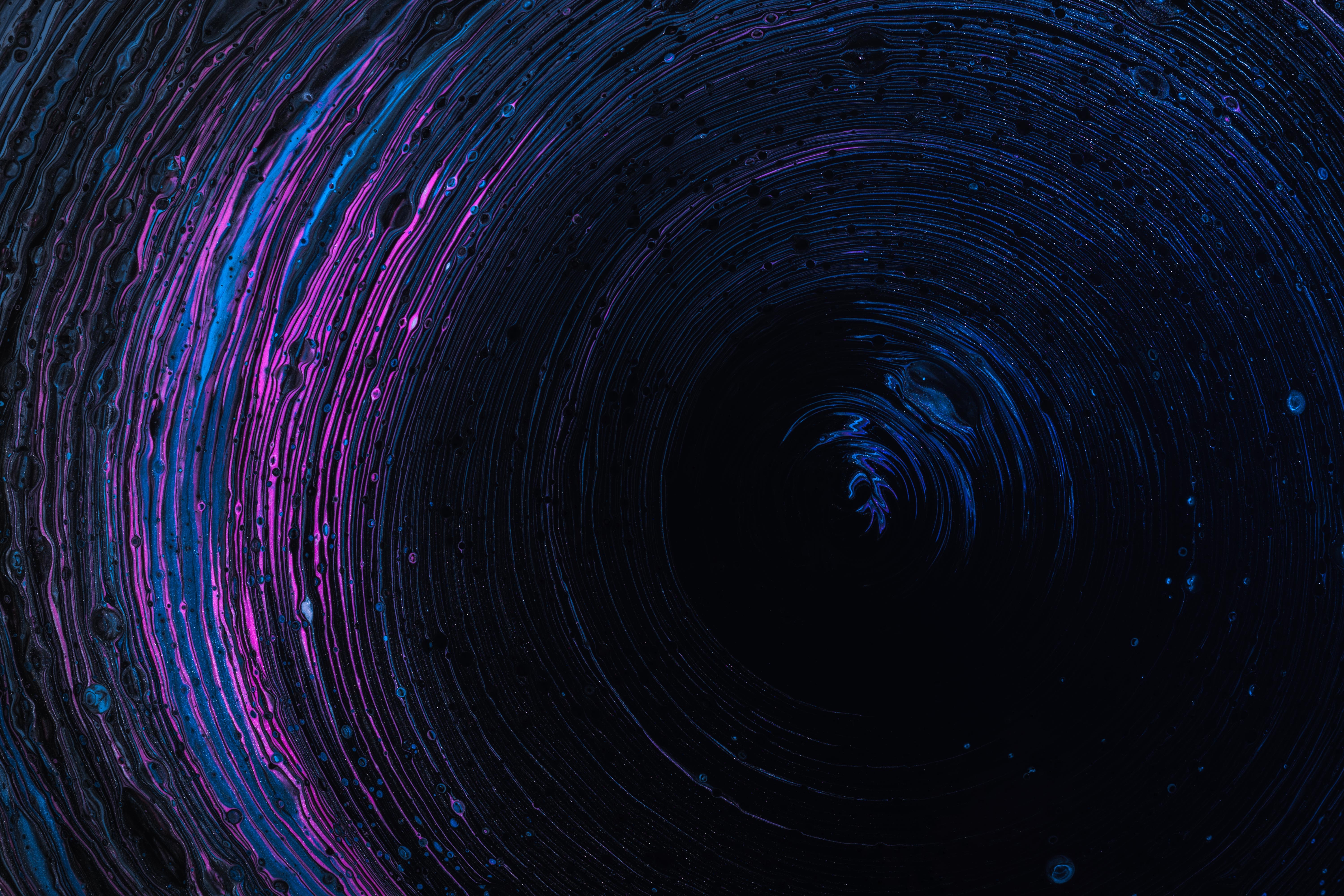
Before performing a market study to assess the commercial viability of your envisioned AI solution, it is important to first perform some crucial data management tasks to make sure that it is possible to build your AI solution, not only from a technology perspective but also from a data perspective. Let’s zoom in on the latter and discuss why it is important to:
- obtain a clear view of the data requirements and availability,
- and build a sound data management strategy in a very early stage of your company.
Data Requirements Analysis
The purpose of every AI solution is to solve a problem. Therefore, the first step is to scope the problem and its context. Get a comprehensive understanding of technologies and techniques for AI solution development.
This will help you understand the data requirements to build your AI solution in terms of required data volume, type, and quality.
Data Gap Analysis
After scoping the problem and its context and obtaining a clear view of the required data to build your AI solution with, it's time to take the next step: perform a gap analysis to obtain more information about the gap between the required data (quality) and the actual available data (quality).
Here's a set of key questions you need to find an answer to before moving on:
- Can you, in one way or another, acquire the data you need? Is the data publically available, do you need to buy it from a commercial provider or should/can you collect or create the data yourself?
- If the data is publically or commercially available: is there a way to assess the quality?
- If you collect or create the data yourself, to what extent can you control the quality of this process?
- Can you improve the data quality via post-processing or by using better technologies?
- What are the ethical, technological, or legal limitations to obtaining and/or using the data and improving its quality?
Data Management Strategy
When you finish the data gap analysis, you should have sufficient information to decide whether or not it is possible to build your AI solution from a data perspective. Even if everything is in your favor, there is still one important task left to execute: building a solid and future-proof data management strategy.
It is important to pay sufficient attention to this task in a very early stage of your company, as it will be the backbone of your AI solution and, by extension, your company. It is crucial to be extra cautious when making decisions at this point. The consequences of making the wrong choice could lead to significant financial losses or, in the worst-case scenario, the failure of your company.
Data Management at Trensition
During the research before Trensition, it became clear that we were handling significant amounts of unstructured data with varying degrees of quality to construct our AI-based strategic intelligence solution.
In 2018, we performed some tests with sample datasets from a couple of well-known commercial providers of unstructured data. However, it instantly became clear that relying on externally available data to perform trend analysis and extract strategic intelligence was not an option.
The quality of the provided data was insufficient. This experience taught us to be cautious of data providers emphasizing data volume but neglecting data quality.
The realization led us to the decision to choose “the dirt road” and start building all our required datasets from scratch. But this, of course, came with its challenges:
- Between late 2019 and mid-2023, nearly every day of the year, weekends included, we spent more than two hours per day creating and managing our own datasets. If you decide to do the same, be prepared to go all in to get the job done. In the beginning, we moved slowly. Which sometimes caused us to be frustrated. However, month after month, we noticed that our patience and dedication gradually started paying off. We were able to offer more, better, and deeper insights to our customers.
- We took full control of the data and decided to set very ambitious long-term goals in terms of quantity and quality. We then built a solid data management strategy and procedures to reach our goals. This method allowed us to keep going for years. Since we highly value qualitative data, we have no intention of compromising our standards.
- We obtained a wide and deep understanding of all characteristics of our datasets and the processes to create, maintain, and grow them. The pain and suffering that we experienced for months, or even years, led us to become masters in identifying and analyzing all opportunities and risks related to the automation of certain data management tasks. The fact that we were also extremely motivated and determined to get rid of the manual labor, helped to keep going.
- In four years, we automated all data (quality) management tasks with a small team of 5-6 engineers and a limited budget. We were forced to become a lean and mean machine, and we still are after all those years. We realized that we had to embrace manual labor to understand how data management can be automated. Speaking of automating data management, we are currently in the process of filing our first patent application.
Looking back at our journey thus far, I dare to state that the path we chose to walk on was the only possible path for Trensition to increase the probability of success of our company in the long term. Sticking to the plan made it possible to celebrate certain milestones:
- Our document database grew from hundreds of thousands of documents in late 2019 to hundreds of millions of documents in mid-2023. That's a 1000-fold increase. As a result of some recent developments, we will likely scale again by a factor of 10 by the end of 2024.
- Since late 2019, our trend database has grown exponentially from 100 to over 2000 trends by mid-2023, which is a 20-fold increase. Thanks to our recent internal research projects exploring the potential of generative AI, we are looking forward to scaling this up to tens of thousands of trends in the near future.
- We found suboptimal but relatively simple solutions for some hard NLP problems, eliminating the need to build complex models that take ages to calculate and only offer a marginal improvement.
A lot of minor and major mistakes were made before the successes, but we learned how to minimize their negative impact.
For example, because of a lack of experience, we underestimated the time and cost needed to build and manage the large data volumes we are currently dealing with. It posed some serious challenges in terms of required human labor for management and computational resources for storage and processing. But up to now, we have been able to scale our systems in a cost and time-efficient way and automate the most costly and time-demanding data management tasks.
We realize that scaling our systems will remain a challenge in the future. If we want to continue handling more data and improve the insights provided on our strategic intelligence platform, we will need to keep our innovative mindset to find relatively simple solutions for complex problems.
It also means that we must better anticipate what's to come and focus more on data management than we already do today.
Takeaways
First data and then solutions. As an early-stage AI company, it's important to put data (quality) management at your company's core. Dare to make bold decisions when building your data management strategy and anticipate the future, even if it means that you will temporarily move slower than your competitors who decided to take the fast and easy lane. Faster rarely equals better, certainly not in AI, where qualitative data is key.
First focus on data and then, and only then, start building your solutions
Don't try to automate data management tasks from the start just for the sake of automation. Be sure that you first fully understand the data and the related processes.
Building valuable AI solutions for your customers implicitly means that you will also have to build AI solutions for yourself to automate (part of your) data management tasks.